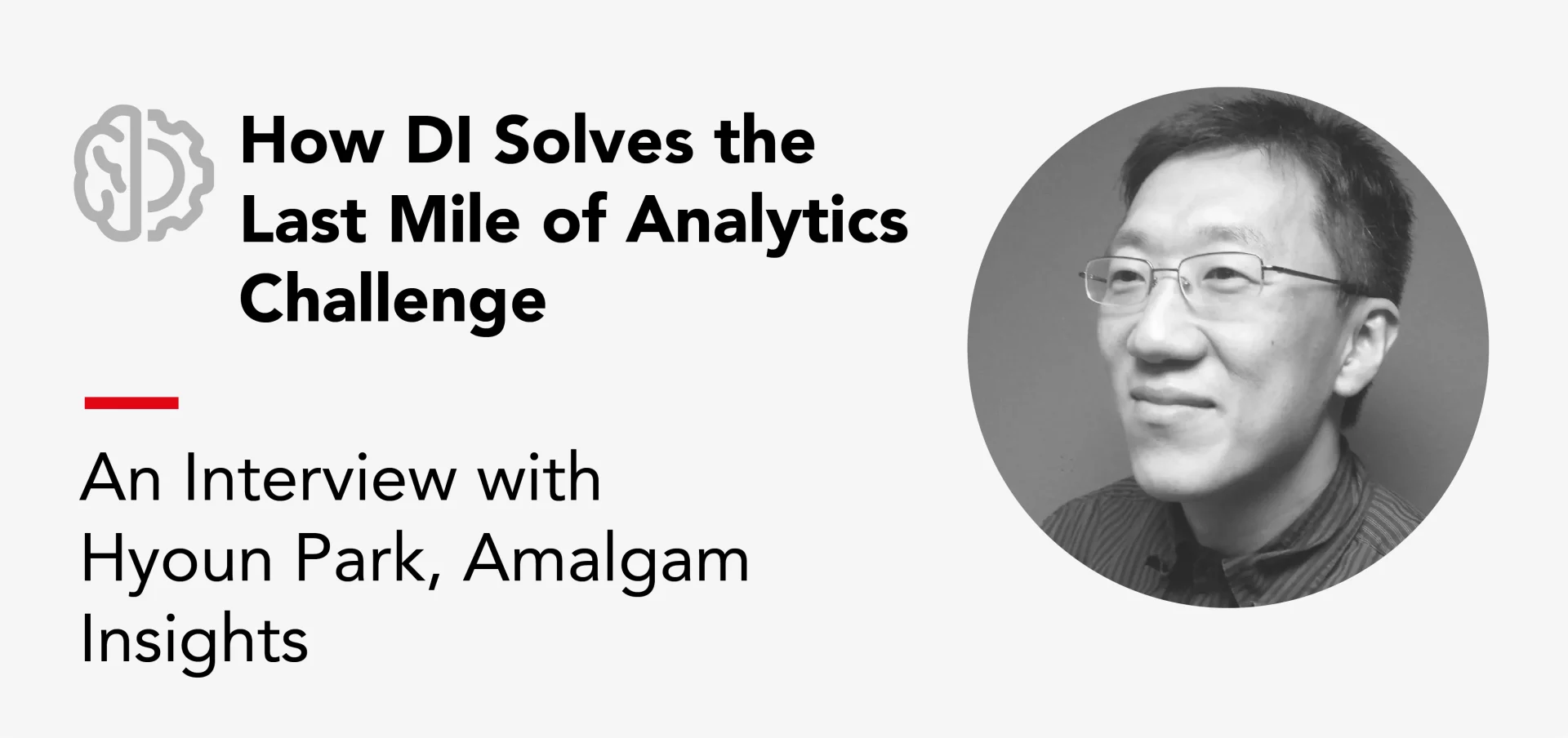
May 9, 2022
How DI Solves the Last Mile of Analytics Challenge
We recently had an opportunity to sit with Hyoun Park, CEO and Principal Analyst at Amalgam Insights, to gather his thoughts on the major challenges facing data analytics in the enterprise. We focused on the role of decision intelligence in closing the “last mile” gap between current technologies and the value large organizations seek to extract from their data analytics investments.
1. Can decision intelligence solve the last mile of analytics challenge?
We live in a world where there is so much data available, and it is growing non-stop. By 2025, there will be ~ 128 zbs of data. The average employee today is simultaneously engulfed by data and yet unable to find the most important data to make the best decisions in real time. So businesses have data, but lack insights to efficiently bridge the gap from data to decisions—what is commonly referred to as the “last mile of analytics challenge.” Companies won’t realize the full promise of data analytics until they operationalize the “last mile” step where insights become actionable. The path to ROI with analytics is better decisions, not better dashboards.
Across industries, digitizing business processes is the norm. Increasingly, human decision-making is unable to keep up with the pace of digital business and the amount of data powering it. Winners in the digital economy will be the organizations that are able to gain insights from their troves of data and drive action. As digitization accelerates, more processes rely on fresh data to function, and decision-making is not immune to this requirement. The challenge is to find a way to systematically convert all the data a company harbors into decisions that unlock growth and support the real-time nature of business today. Decision intelligence (DI) helps operationalize decision-making, making it more reliable and consistent with the ability to quantify the business impact of decisions. It also supports the kind of workflow people need in today’s world—more exploratory, interactive and diagnostic—and where decision-making happens more frequently and in real time.
“Decision intelligence (DI) is the ability to use relevant and available data to identify and explain optimal actions that improve business outcomes.”
Decision intelligence is uniquely able to solve the final step in the analytics process. This is the step in which insights are translated into outcomes that a frontline worker or manager can use to drive business value. In fact, I believe DI will be the most important value driver for enterprise analytics and machine learning this decade and will be fundamental to solving the last-mile problem.
Decision intelligence does this by automating insight production and producing explainable, quantifiable and actionable recommendations to augment human decision-making. DI delivers a real-time understanding of important metrics, in turn helping a user to see not only what is happening in the business but also what can be done to improve the outcome. From a practical perspective this means bringing together the data, metadata, analysis and machine learning capabilities needed to create a system that recommends the best action to achieve a goal and then dynamically updating next best actions as the situational context changes.
Challenges to translating analytics into action inevitably come down to the people who use analytics to make decisions. Another important dimension of the last-mile challenge is the UX for consuming analytic insight. Until recently, business users have depended on static tables, graphs in reports, or dashboards to understand how their analytics team came to a conclusion. If they have a question about the results, it’s time-consuming to get the analytics team to respond, and the requested insights are often stale by the time they reach a decision-maker. In the digital era, decision-makers need to understand what’s happening in real time as well as easily explore data and results, see what would happen if they changed something about the input parameters or the assumption, and see how that would affect the results of the analysis. Providing recommendations with visibility into the impact of acting on them, makes it easier for a user to swiftly and confidently decide.
“ With decision intelligence, business users no longer have to wait on data analysts or data science teams to get answers fast.”
Finally, there is the lack of systemic assessment and feedback on the success of decisions, which prevents users from learning and improving the way they make decisions. DI incorporates the capacity to monitor and improve its decision-making abilities based on human feedback and by comparing the actual outcomes of decisions with the predicted one to improve the system going forward.
When a business provides decision-makers at all levels with decision intelligence while simultaneously creating accountability to use it in the decision-making processes, then it is possible to deliver meaningful reductions in the data-decision gap and conquer the last mile of analytics. Given the trend to digitize business processes and the pivotal role of decision-making across every organization, it’s a question of when businesses will deploy decision intelligence to close the decision gap and truly enable pervasive data-driven decision-making.
2. How can decision intelligence help more people improve decision-making in the daily routine of their jobs?
Decision intelligence augments human decision-making. It eliminates barriers for people with business context to leverage advanced analytics by connecting data, decisions, actions and outcomes into a streamlined and automated process.
Until now, using AI, ML and other statistical methods to solve business problems was mostly the domain of data scientists. New tools like decision intelligence that combine the insight and power of AI and ML with a decision-oriented workflow, make analytics more consumable by a broader population of users (non-data scientists). The proximity of domain experts to the unique problems their organizations face, the metrics they monitor and their specialized knowledge about their line of business, give them an advantage in validating AI-powered recommendations and decision-making. Where they may have relied on their gut or intuition, they can now use their experience in conjunction with AI-powered insight to make better decisions, faster.
We are at the beginning of exploring what decision intelligence capabilities in the hands of people closely aligned with the business will unlock. Decision intelligence is an opportunity to improve human judgment by enhancing it with appropriate data, not a chore forcing users to constantly train a system with relevant information in today’s fast-changing work environment. When enterprises empower everyone—across all levels and roles—with access to contextual business insights and actionable AI-powered recommendations, they will no doubt realize a measured improvement in operational efficiency, increased profits and overall competitiveness. Together, human expertise, judgment and contextual awareness combined with the rigor, automation and scalability of AI-powered insights and recommendations will be a powerful accelerant of competitive advantage in the digital era.
3. Where should decision intelligence be used?
To deal with unprecedented levels of business complexity and uncertainty, organizations must make accurate and highly contextualized decisions more quickly and frequently. With more data than ever before, the ability to quickly answer questions about both what’s happening and why in time to act is a challenge. Businesses with decision intelligence systems hold a competitive advantage since DI can sift through high volumes of data quickly, accurately and often with minimal or no human intervention. Decision intelligence is ideal for companies with vast data stores and teams of business analysts and data scientists who are overburdened with large-scale, highly repetitive, day-to-day operational reporting requests. It’s also useful for decision augmentation for strategic, tactical and operational matters.
Deciding where to begin using decision intelligence will depend on the complexity and frequency of the decisions. The more straightforward and repetitive a decision, the more easily it can be automated and the more value can be unlocked by augmenting human decision-making. The value of DI is the operational advantage that results from front-line business users and executives being able to rapidly ask and answer key business questions like “Are we meeting our daily KPIs?” or “Is there a drop in our conversion rate and what can I do about it?—and then being able to act in real time.
For example, DI supports decision-makers in regularly or consistently recurring decision scenarios, like inventory optimization. In this example, DI leverages SKU-level demand forecasting in order to make accurate inventory planning across a supply chain and identify outages ahead of time in order for proper adjustments and action. Often this type of analysis involves techniques such as root cause analysis, key driver identification, scenario or what-if-analysis that happens regularly. Operationalizing decision intelligence in this use case results in significant cost savings and efficiency gains and frees the business user up from repeated tasks. Using decision intelligence, line of business users can reach the best possible data-driven business outcomes in the shortest time possible and reduce their dependence on “gut” interpretation of insights and dashboards.
4. How does decision intelligence fit into the modern data stack?
The modern data stack is built with modern (and more types of) data sources in mind. Tools of the modern data stack are individually trying to solve for speed, complexity and scalability, ultimately shortening the time between analyzing data, decision-making and the business value derived from it. Because the modern data stack is highly automated, it provides the potential for reimagining entire analytics processes, leading to much fresher data and quicker time to insight.
Decision intelligence integrates into the modern data stack at the top level to enable a new set of capabilities to accelerate decision-making. It takes all of these different pieces and allows them to work together from an infrastructure perspective to optimize the user experience.
In its simplest form, a modern data stack encompasses an ingestion tool, a warehousing tool, a transformation tool, analysis and model building tools and the “decision” layer built on the advanced analytical capabilities of decision intelligence. Each of these layers play a key role in an organization’s ability to get actionable insight from vast amounts of data and ML models, develop recommendations for action and accelerate decision-making.
……
The “last mile” challenge in data analytics will require the application of technologies that simultaneously automate and streamline analytic tasks across vast amounts of data while giving business decision makers actionable insights and rapid answers to alternative scenarios. Decision intelligence delivers a faster, more reliable time-to-decision (compared to legacy BI tools) based on its use of AI, machine learning, contextual intelligence, process automation and UX innovation. Decision intelligence is an emerging tool that can make this possible, provided it integrates with an organization’s existing data stacks, data science investments and workflows.