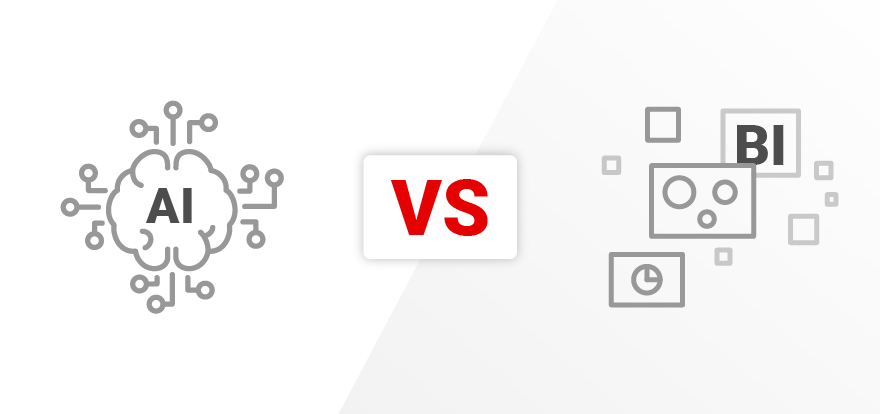
March 26, 2021
Why AI Models Eat BI Dashboards for Lunch
Many organizations devote precious time and resources developing predictive AI/ML models to determine what might happen in the future. The ultimate goal of these models is to use data to inform decision makers about the next best action. All too often, these models end up in business intelligence (BI) and visualization tools, and displayed to the end user via dashboards.
This approach is problematic because it wastes the knowledge potential of machine learning (ML) models. Dashboards are designed to inform the business user about what’s happening on a near-term basis, and don’t necessarily help them make better decisions, or even connect the dots between what’s happening in other areas of the business.
Here are a few reasons why dashboards and ML models don’t mix well, and our suggestions for a better approach via decision intelligence.
Dashboards still require manual analysis
Let’s look at an example to understand exactly what a user might see with a predictive forecasting model embedded in a dashboard. If you’re an inventory analyst at a home improvement retailer, you may see via your dashboard that this year promises to be a hot summer. You could use a separate dashboard to view your inventory plans for your merchandise; for example, the products you intend to stock, when you stock them, and where there may be gaps.
In this scenario, it’s still on the inventory analyst to decide exactly how much more of each product to order. For example, they may decide that a hot summer means people do more gardening. As a result they may stock up on potting soil, watering cans, plants and more.
The probability that they’re making a right decision relies on the analyst understanding correlations among the observed trends and patterns, analyzing the data in dashboards to determine root-cause, quantifying their decision’s impact, and ultimately contextualizing the resulting numbers for the rest of the business.
This process is difficult, time-consuming and manual – which means it’s not scalable. Also, depending on the experience and judgment of each analyst, it tends to introduce human bias into the picture.
AI models ingested by dashboards can’t connect the dots
One of the biggest shortfalls of combining dashboards and ML models is that dashboards cannot make actual recommendations that impact multiple parts of the business. In the example above, the analyst only has visibility into their area of the business: inventory management. As a result, it’s hard for them to instantly understand the impact of their decision on adjacent areas of the business, including finance, logistical operations and more.
What’s more, information can change quickly across departments. Analysts only have so much capacity to sit down and do a deep dive into their dashboards on a daily basis. It can also be difficult to use the output of one model in reference to another, in order to explore “what if” scenarios. In our hot summer example above, perhaps the analyst’s decision to purchase further gardening inventory could result in disproportionately negative effects on other product lines. With dashboards, it’s impossible to tie the outputs of two models together to visualize the effects of one decision on another.
A decision intelligence system can take this burden off of analysts by automatically ingesting data from multiple ML models, making connections between relevant data points across departments and functions, and providing real-time recommendations on the next best actions for the business.
Moving from operational to tactical decisions
Predictive models as they’re used today are great for low-level, or single-step operational decisions. For example, a credit fraud model could predict an instance of potential fraud and trigger an action, such as an email to the cardholder. However, with current business intelligence systems, it’s still difficult to translate that value to tactical decisions that require interrogating multiple ML models and synthesizing data from many different sources in order to make a decision.
The inventory example above is a tactical decision that requires an understanding of budget, consumer behaviors, supply chain management, profitability impacts, and more. For example, if the inventory manager stocks up on low-margin barbecue equipment due to the hotter weather, how will this decision affect profitability?
A decision intelligence system could help analysts understand these impacts instantly. The system can also adapt and learn from human feedback, so that decisions are fine-tuned and become more accurate over time.
Decision intelligence systems like Diwo can help realize the full potential of your AI/ML models. Want to learn more?