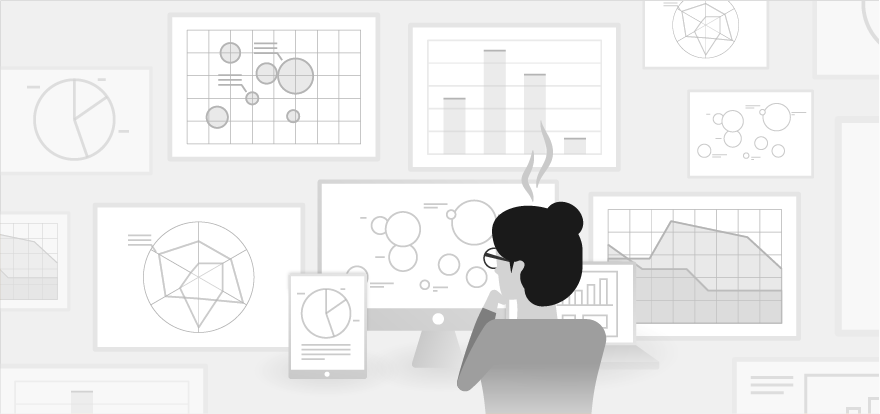
March 17, 2021
Why More BI Dashboards Don’t Mean Better Decisions
A typical analytics dashboard, whether it’s viewed as a pivot table in Excel or within a business intelligence (BI) platform, is often the primary source for data-driven decision-making. Analysts spend hours and hours in dashboards – looking for patterns in the data to draw meaningful conclusions. However, there’s one big problem with dashboards: They were engineered to inform, not prescribe actions in response to business moments and events.
Here are three reasons why dashboards don’t equal better business decisions, and how to take a more modern approach using decision intelligence.
Self-service BI delivers fragmented insights
Often the insights derived from self-service BI doesn’t result in actionable next steps. Dashboards generally deliver fragmented insights. For example, an analyst may navigate multiple dashboards, collecting insights from each in order to arrive at a decision on their own.
In other words, there’s no easy way to ask a question and get an answer within a dashboard. Most of the time is spent in dashboards doing ad-hoc, manual analysis or manipulating data to fit a predetermined conclusion or thesis, with the results transferred to spreadsheets for analysis. It’s often a labor-intensive process that relies on teams of analysts to synthesize both historical and predictive insights, analyze root cause, quantify impact, and ultimately contextualize the best results for business consumption. Not only is this process complex and time-consuming, but its manual nature introduces subjectivity and bias.
BI dashboards are anchored in the past
Perhaps one of the biggest problems with dashboards is that they’re fundamentally designed to analyze historical data. Predictive information, on the other hand, is more closely tied to action. As a result, predicting what will happen in the next quarter (or for any designated time period, really) is just an exercise in projecting out historical trends. Any true tactical decisions still require a high degree of human (or analyst) intervention.
Many machine learning models built for predictive decisioning don’t work well within historically oriented dashboards. For example, if a very accurate forecast is represented as just another data point on a chart, what real value did the forecast deliver? Chances are, the decision-maker’s behavior didn’t change significantly. And it’s ultimately up to them on how they want to best interpret and execute against these data points. The real business value lies in creating recommendations out of these predictions, instead of just charting them on another dashboard.
They lack context for decisions
Business context is one of the biggest missing pieces when it comes to dashboards. That’s where they fall flat in providing fuel for recommendations on the next best action. Dashboards were built to visualize data; for example, how much of your inventory is left on shelves at the end of the current season. However, this information doesn’t automatically translate into what to do with that inventory or help you anticipate what to do next year as retail stores reopen globally.
With so many possible scenarios to model, analytics can be difficult to consume – not to mention, it’s even harder to land upon a relevant outcome when faced with a potentially irrelevant data set.
As stated in this Gartner Top Trends in Data and Analytics for 2020 blog post, “Dynamic data stories with more automated and consumerized experiences will replace visual, point-and-click authoring and exploration. As a result, the amount of time users spend using predefined dashboards will decline. The shift to in-context data stories means that the most relevant insights will stream to each user based on their context, role or use.”
The shift to decision intelligence
Many organizations may continue to use dashboards for historical analysis, but augment this technology with an emerging practice called decision intelligence. The same Gartner blog referenced above notes that “By 2023, more than 33% of large organizations will have analysts practicing decision intelligence, including decision modeling.”
Decision intelligence technology uses AI to augment a human’s decision-making process. The goal is to understand and apply users’ context to data, helping to predict opportunities for growth and minimize risk. In addition, this technology can recommend the best strategies to address potential issues and quantify their impact. Rather than spending hours in dashboards, decision intelligence helps analysts quickly model different scenarios so business teams can make faster decisions.
Does your team still rely on dashboards for decision-making? Reach out to us to learn more about how decision intelligence is different.