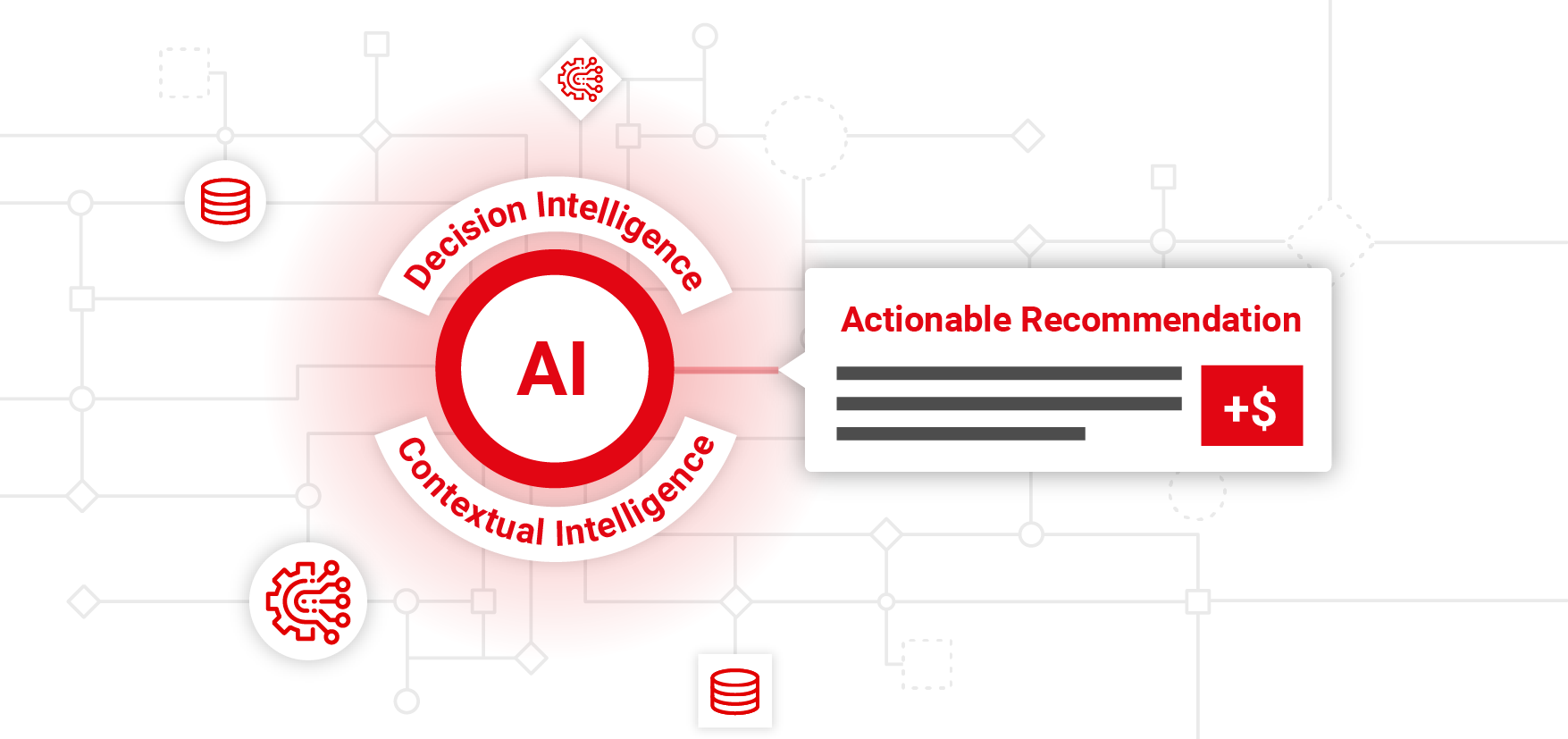
March 22, 2022
The Decision Intelligence Data & Analytics Tech Stack
In the past two decades, enterprises have made massive investments in BI and visualization tools, data lakes, and machine learning platforms to deliver data-driven insights and optimize business outcomes. Despite the tsunami of dashboards being produced, effectively turning dashboards and insights into business value remains elusive. The “last mile of analytics” – the final step in the analytics process in which humans must translate dashboards into outcomes that drive value – is still a challenge for many organizations.
Decision intelligence (DI) leverages AI and Contextual Intelligence to create output in the form of specific and actionable recommendations instead of dashboards and reports. This advanced application of AI means that data-driven decisions are efficiently, consistently, and rapidly enabled for suitable use cases. Rather than searching for insights, business users evaluate a DI-driven recommendation and make decisions with high accuracy, frequency, and absence of human bias.
Decision Intelligence is producing a paradigm shift in analytics. It is predicted that the decision intelligence market will reach $17B by 2027. Today, the visualization market is 15+ years old and stands at only $17B. Gartner predicted 33% of enterprises will deploy DI by the end of 2023.
Making Sense of the New Analytics
When paradigm shifts occur, confusion is usually present early in the evolution, which is true today with decision intelligence. Many analytics vendors hop on the decision intelligence bandwagon without having the appropriate technology. So we must clarify the three types of advanced analytics intelligence tools available today.
Business Intelligence – These are the most adopted analytic tools on the planet and have been around for 25+ years. The category starts with the SAS and MicroStrategy tools and includes more recent stalwarts such as Tableau, Power Bi, Looker, and Qlik. These legacy vendors generate reports, charts, graphs, and the omnipresent dashboard.
Augmented Intelligence/Automated Insight– A more recent advancement in the analytics space is to leverage AI to uncover and surface insights from data, bypassing the inefficient chore of users trying to analyze a myriad of dashboards and manually surfacing insights. While certainly better than a dashboard – but like its predecessors in BI – augmented intelligence tools are still in the business of producing insights, not consuming them. Current tools in the category include: Thoughtspot, Sisu, Tellius, Sisense, Domo, and Peak.ai[DC1].
Decision Intelligence – Decision intelligence uses AI to surface insights, similar to augmented intelligence. However, decision intelligence then consumes the insights surfaced to deliver specific and actionable recommendations. DI platforms provide insights, areas of improvement, and the recommended course of action as the output. Producing recommendations, often with the assistance of contextual intelligence, is core to decision intelligence and separates actual DI platforms from the pretenders[DC2].
Decision intelligence will replace business intelligence, right? Not exactly. Decision intelligence is more suited to frequent and repetitive decisions, such as upsell target customers, preventive maintenance, inventory allocation, purchasing, pricing, etc. BI will likely still be required for reporting and research activities for decades to come.
No Excuses, No Re-Engineering Required
The most significant advantage to decision intelligence may lie in its ability to deliver advanced recommendations by using the existing architecture that an enterprise has spent years building at a very high cost. Decision intelligence will leverage whatever data fabric, data warehouse, or data source that currently exists to support BI. Furthermore, DI will also leverage any and all AI and M\L models that an enterprise has developed. This means minimal impact on the enterprise’s data engineering and data science resources to implement decision intelligence.
Let’s review the stack itself.
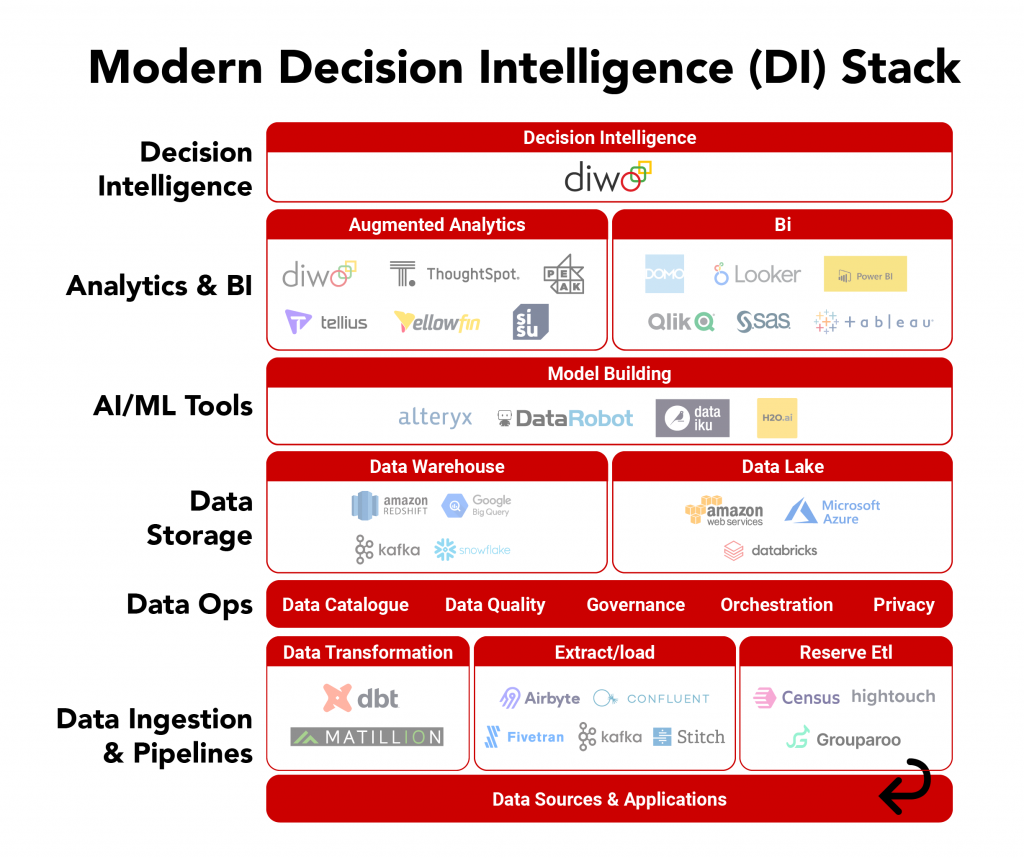
Data Sources
Not much has changed in the components of the first few layers of the Data & Analytics tech stack. The technology has been relatively static, with the most significant impact being moving from on-premise to the cloud. But whether the applications that create data are in your data center or in AWS, they still serve as the bedrock for every data architecture.
Integration, Transformation, and Data Ops
As the data moves up the stack, the traditional ETL has been replaced by ELT and augmented by Reverse ELT tools. There have been substantial advancements in data integration, rendering what once was an arduous process into something very feasible and quite manageable today. Governance, Lineage, Quality, and Security continue to be core elements of the quixotic quest to “get our data right.” It is a never-ending pursuit, and most have accepted that they can pursue value from their data without waiting for it to be perfect.
Storing Data for Decision Intelligence
Repositories for analytics-ready data have changed significantly in the last five years with great hype, fanfare, and investment. The shift to the cloud is ongoing, and platforms like Snowflake stand to benefit from this continued migration. What already resides in the cloud is still just the tip of the iceberg in terms of total data. One of the more exciting advancements is the creation of data fabrics or, in the case of Starburst, a data mesh to provide access and governance without relocating the data.
It is important to note that, like BI tools, DI platforms can pull data from their original data sources if required. However, optimal performance often dictates that data reside in a repository design to support analytics.
Data Science and Machine Learning
The data science layer has moved from the automation of model creation to the orchestration of models and has seen significant consolidation of vendors. Current advances are making codeless model creation possible as well as orchestration of hundreds of models within an enterprise. This part of the tech stack is critical for decision intelligence because nearly all DI use cases require models. If the necessary models do not already exist, they will need to be developed and accessed by the DI platform.
Production of Insights
Finally, the Business Intelligence and Automated insight layers produce visualizations of the analytic output in the form of dashboards or directly surface insights. Modern data analytics include tools to help non-technical users explore data without knowing SQL. This frees them from depending on developers and analysts and encourages everyone to explore and learn from data. These tools could provide their output to a DI platform, but the automated analytics process frequently stops here, and a very inefficient manual cognitive process takes over.
The Decision Intelligence Data & Analytics tech stack is a prescription for resolving the Last Mile of Analytics challenge[DC3]. By leveraging all the previous investments in data engineering and data science, a decision intelligence platform will consume insights and produce actionable recommendations that create immediate business value. Download your copy of the Decision Intelligence Tech Stack, and If you are ready to evolve beyond BI dashboards and get to better decisions faster, read the solution brief Getting Started with Decision Intelligence.