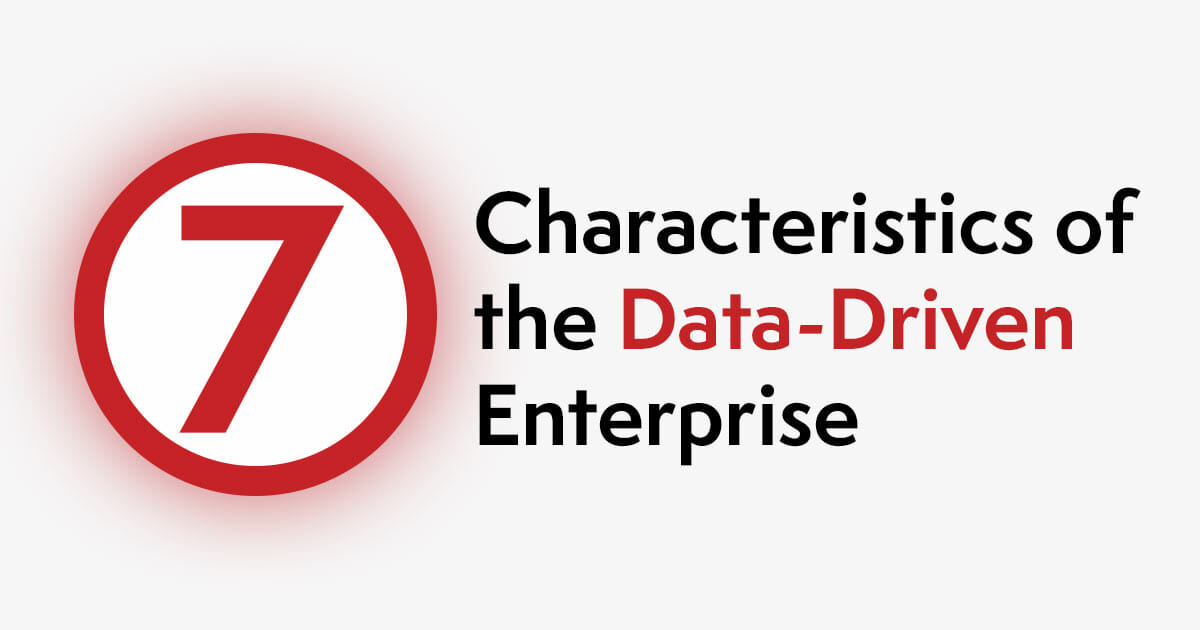
August 16, 2022
McKinsey’s 7 Characteristics of the Data-Driven Enterprise
Rapidly accelerating technology advances, the recognized value of data, and increasing data literacy are changing what it means to be data-driven – so why is there still a gap between data and decision-making?
Even as the wealth of available data continues to grow, enterprises today still miss out on the opportunity to use data to improve decision-making, influence key metrics, and drive impact. The majority of organizations still struggle to identify what happened, let alone why it happened and what actions they should take next. On top of that many companies don’t have enough context around their data to make confident decisions or hypotheses.
Despite the amount of data available today and the rapid evolution of modern data infrastructure, how decision-makers actually put data to use – the last mile of the data lifecycle – hasn’t changed much.
The BI tools that remain in widespread enterprise use were designed to be descriptive (what happened) rather than predictive (what’s likely to happen next). They’re rarely prescriptive (telling you what to do). Data scientists do have access to modeling tools that are predictive in nature, but data scientists are a scarce (yet valuable) resource in most enterprises. Most are consulted relatively infrequently because data science requires time-consuming work, which may not fit within the business’ timeline or resources.
Closing the Gap Between Data and Decisions is Essential to Becoming a Data-Driven Enterprise
According to a survey by McKinsey, decision-making processes tend to be time-consuming and ineffective. In fact, only 20% of respondents to a recent survey of leaders conducted by McKinsey said that their organizations excel at decision-making. Just 37% said that their organizations’ decisions were both high quality and high velocity. Further research from McKinsey shows that data-driven organizations are 23X more likely to acquire new customers, 6X as likely to retain existing customers, and 19X more likely to be profitable.
In a world where making good decisions is vital to every enterprise’s success, it’s essential to close the gap between data and decisions. Many businesses today are deploying new data-driven strategies and models to promote growth, accelerate digital transformation and increase operational efficiency. However, to maximize success, enterprise leaders must incorporate decision intelligence which delivers data-driven insights and recommendations into their decision-making processes. Decision intelligence augments human decision-making with AI/ML to generate faster, better insights and actionable recommendations from enterprise data.
Key Take Away from McKinsey’s Data Driven Enterprise of 2025 Study
According to a recent McKinsey study – The Data-Driven Enterprise of 2025 – smart workflows and seamless interactions among humans and machines will likely be as standard as the corporate balance sheet and most employees will use data to optimize nearly every aspect of their work. In this study, McKinsey outlines seven characteristics* that will define the new data-driven enterprise.
#1 = Data is embedded in every decision, interaction and process.
By 2025 Nearly all employees naturally and regularly leverage data to support their work. Rather than defaulting to solving problems by developing lengthy – sometimes multiyear – road maps, they are empowered to ask how innovative data techniques could resolve challenges in hours, days, or weeks. Organizations are capable of better decision-making as well as automating basic day-to-day activities and regularly occurring decisions. Employees are free to focus on more “human” domains, such as innovation, collaboration, and communication. The data-driven culture fosters continuous performance improvement to create truly differentiated customer and employee experiences and to enable the growth of sophisticated new applications that aren’t widely available today.
Decision Intelligence Provides the Foundation for Data-driven Decision-making
Modern data strategies are incomplete without robust analytics capabilities that produce timely, proactive and granular insight as well as actionable recommendations. Decision-makers today need context, real-time intelligence and automated tools that draw out the “why” and “how” behind what’s happening in the business. And they need these capabilities packaged in a way that allows all business stakeholders to utilize them in their day-to-day activities.
With decision intelligence, it’s now possible to build an organization where any decision can be evidence-based and informed by data and where it’s possible to take decisive and effective action at speed. Decision intelligence can guide decision-making by supplying AI and ML powered analytics that quickly reveal what matters most and recommendations about what to do next. This increases data analyst efficiency and gives decision-makers access to fast, contextual insights and actionable recommendations. It makes it possible to monitor KPIs, investigate changes, and gain visibility across all relevant business factors – without specialized assistance from the data team. The more people who can access data, ask questions of data and iterate on insights, the better decision-making becomes in the organization.
When you combine technology with processes that enable people to put decision intelligence to work in their day-to-day job, you’ve got a recipe for actionable data-driven decision-making – at the speed and scale of the modern enterprise. With cutting-edge analytic solutions that put decision intelligence at the fingertips of stakeholders across the business, it’ll be easier than ever to unleash the full potential of data, make better decisions and create new sources of value.
* The following are the seven characteristics of the data-driven enterprise: 1. Data is embedded in every decision, interaction, and process. 2. Data is processed and delivered in real time. 3. Flexible data stores enable integrated, ready-to-use data. 4. Data operating model treats data like a product. 5. The chief data officer’s role is expanded to generate value. 6. Data-ecosystem memberships are the norm. 7. Data management is prioritized and automated for privacy, security, and resiliency.