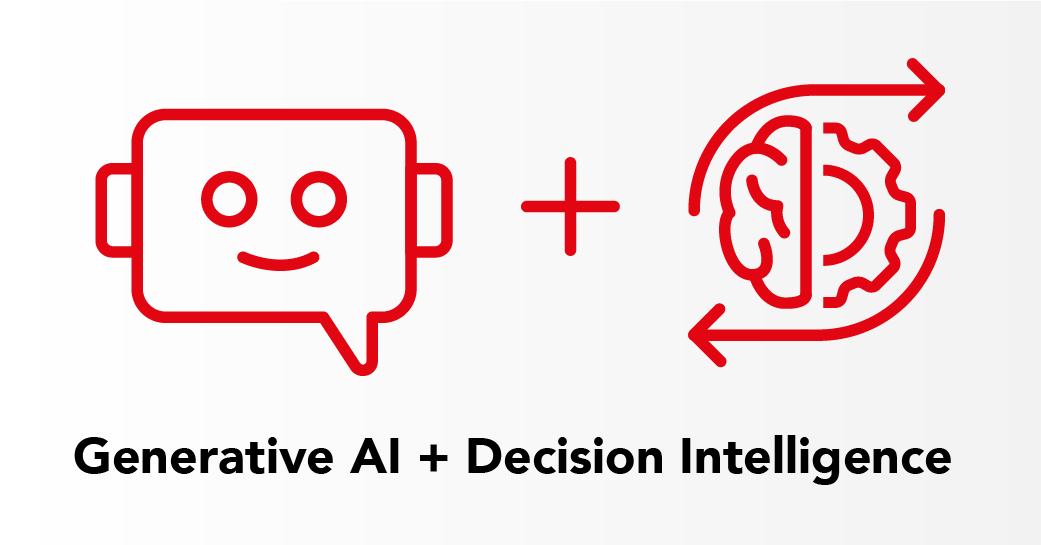
How can Generative AI and Decision Intelligence enhance each other’s capabilities?
With the unprecedented adoption of ChatGPT and now Google’s Bard, many are quickly discovering the potential of Generative AI. However, Decision Intelligence hasn’t captured the attention of the general public in the same way, as its transformative capabilities are currently reserved for more complex business decisions. So what are the potential synergies between generative AI and Decision Intelligence? How can these two different fields of AI with distinct goals and applications add value to each other?
Generative AI refers to a type of AI that is capable of generating new content or data based on or similar to the input it has been trained on. Decision Intelligence is a subfield of AI that focuses on helping humans make better decisions in their own context by combining insights and output from data and advanced analytics. It helps answer the question, “What should I do to achieve my desired outcomes?” DI goes beyond simple data dashboards and visualizations to explain the path from actions to desired outcomes.
Let’s look at what Generative AI or Large Language Models such as GPT can bring to the table.
Research and content generation for Decision Models
As a research assistant, it can be helpful in creating decision elements for a decision model or “causal decision diagrams”- which map the connection between actions and outcomes. This is demonstrated in an article by Dr. Lorien Pratt, in which a dialogue with ChatGPT about Net Zero GHG emissions illustrates how it can help elicit knowledge and suggest potential actions to reduce emissions and travel. An SME is still required to both ask the right questions and curate the answers, so there is absolutely no Decision Intelligence being performed by the Generative AI tool, but rather a very useful collaborator to help create the decision model (more on Prompt Engineering later).
Decision Intelligence output consumption
DI output has generally been in some form of natural language statements, as derived insights are combined and contextualized for the user in the form of straightforward recommendations. LLMs can be informed by DI models to further power the DI transformation with conversational interactions and powerful interactions that inform decision making in the business moment rather than data exploration. It must be said that without a decision model, LLMs on top of any data structure or Analytics/ BI tool is still a form of self-service BI.
Providing synthetic data for ML, augmenting existing data and identifying anomalies
Generative AI can add value by creating synthetic data that can be used to train machine learning models. This can save time and reduce the cost of collecting and labeling large amounts of data. It can also be used to identify anomalies in data that may be difficult to detect with traditional methods. Generative models can learn the patterns and structures of normal data and identify data points that deviate from these patterns.
Creating the semantic layer for context building
A semantic layer serves as a common vocabulary and definitions that provide a standardized view of data sets and insights derived, critical for any enterprise AI solution. It maps the various data sources to a consistent set of terms and definitions, allowing for seamless integration and analysis. One of the most powerful ways to leverage these LLMs for DI is in building and testing the semantic layer. Decision models will inform the relationships for different ontologies and Generative AI can now help automate this process and continually optimize it, which is one of the most critical aspects of any Decision Intelligence platform.
If we look at it from the flipside, what can Decision Intelligence do for Generative AI?
The ultimate ‘query engineer’
Decision Intelligence can be used as a framework for query engineering Generative AI as it provides a structured approach to designing and evaluating generative models. By definition, Decision Intelligence sets objectives, and it can basically do the same for Generative AI models. This helps prioritize the objectives and constraints based on the needs of the business in order to then formulate queries that are relevant to the end-users. The query performance can be further optimized based on the business needs and end-user feedback. The ideal consumption/ interface for Decision Intelligence would reflect a user “decision flow” that provides both quantified recommendations and simulations that would provide decision support when and where the user requires it- which is why we set out to build Diwo. As LLMs are not designed to assist humans in their decision-making process, Generative AI-powered Analytics like the new Azure Open AI service will pose some significant challenges for Analytics consumers. While LLMs offer general knowledge and context from their training, they may struggle to accurately answer specific factual questions with ambiguous queries, limited parameters and external verification. If LLMs can provide answers to questions users may have in the context of a specific step of their decision-making process, the business semantics, context and data set in the “decision flow” can be used as a means to better define parameters. DI methodologies will play a vital role in solving some of the inherent limitations and training methodologies of LLMs.
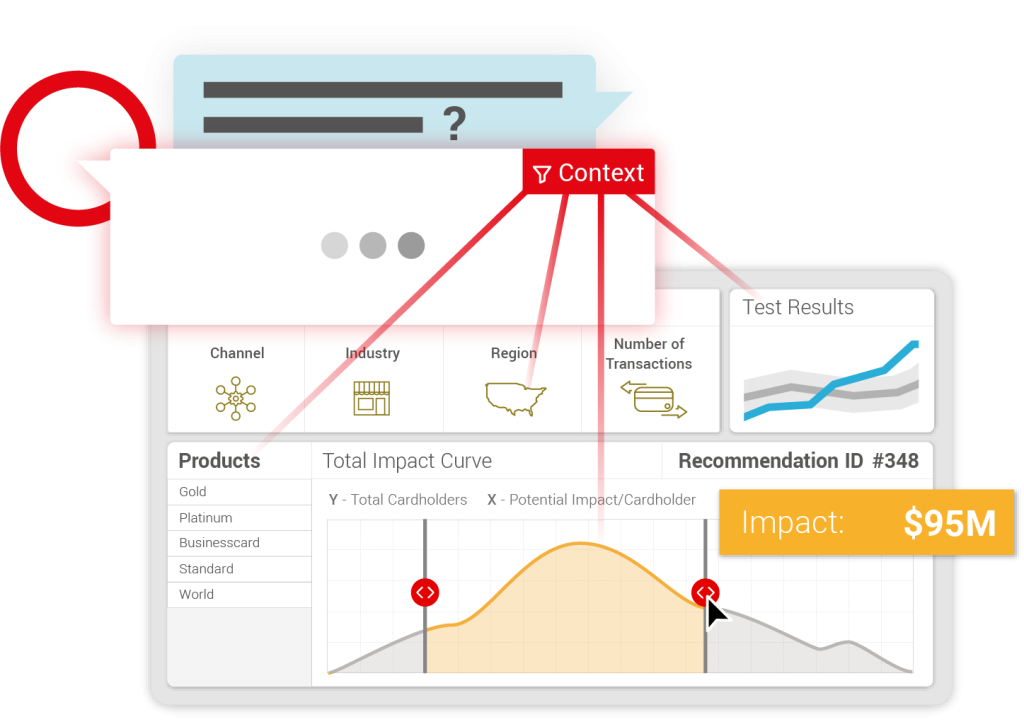
Feedback loop and iteration
DI depends on keeping humans in the loop to learn from their interactions and decisions, user feedback can be used to identify areas where the model needs improvement and to prioritize development efforts. DI techniques can also be applied to Generative AI to improve the quality and efficiency of the generated data, with optimization algorithms that find the best hyperparameters and model architectures for Generative AI models. As feedback and DI models are incorporated into the Generative AI model, these can also be used to identify new applications for the LLM.
The emergence of ChatGPT and similar AI technologies has generated great excitement by expanding the collective awareness about the potential to create increasingly valuable outcomes through AI. The progress in its applications are gaining momentum like never before. For a Decision Intelligence platform like Diwo, it’s exciting to see how much value these two types of AI bring to each other.
Other FAQs:
What are the differences between Generative AI and Decision Intelligence?
How does Decision Intelligence leverage Contextual Intelligence?