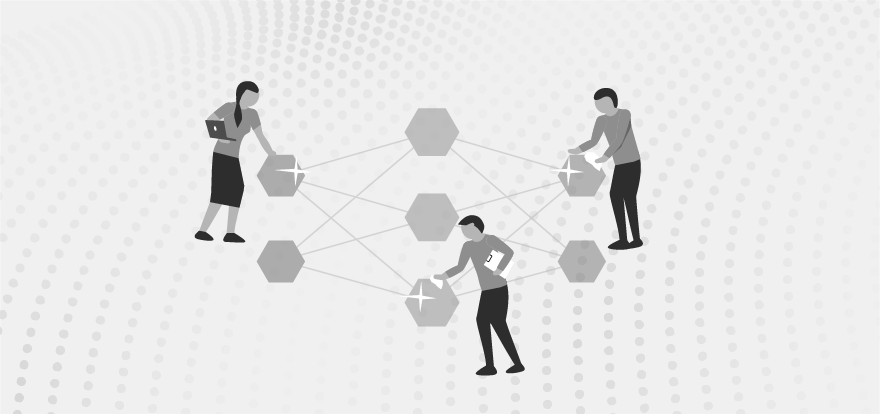
April 22, 2020
Don’t let your shiny AI models lose their luster!
Businesses have made significant investments in building AI/ML models in recent times. While models are increasingly driving significant operational efficiencies and differentiation for businesses, they are often blamed when they fail to predict accurately in extreme events such as COVID-19. In reality, model decay is inevitable: markets are changing quite fast, and trends are short-lived. Black Swan events can create a ‘new normal’, invalidating the history on which models are trained. Organizations typically have an inventory of several hundred models; is it possible to monitor decay proactively and manage interdependencies in a rapidly changing environment?
Once a model has been formally put into use, model decay can begin to occur due to increased uncertainty over time. Data Science teams will have to ensure that continuous monitoring is in place, performance thresholds are set and triggers are defined to ensure that areas of potential risks are highlighted, mitigated, and escalated as necessary. Organizations typically conduct model monitoring on a quarterly/half-yearly/yearly basis based on the material impact. Since drift can occur anytime (due to data and/or model), continuously monitoring and identifying drift proactively can avoid missed opportunities, mitigate loss and enhance trust in the models used. Models are recalibrated when the drift is beyond set tolerance levels. There is no one model that is right for every situation; organizations often have both champion and challenger models, and continuously track their performance and divert traffic to the model that performs best. Challenger models can be deployed in shadow mode to avoid any negative impact on the end-user experience.
The stability of the population and its underlying characteristics must be reviewed when the model is executed in production to avoid data drift, which can be measured by metrics like Population Stability Index (PSI)/Characteristic Stability Index (CSI) and KS statistic. PSI/CSI help to monitor if there is a shift in the population between development and production data. KS statistic is used to assess the predictive capability and performance of the model.
Since trends and relations that exist in the historical data are used to train models, models become obsolete when trends become obsolete or historical correlations are broken. These changes can be gradual (change over time), recurring (cyclical change), or sudden (abrupt change). Model monitoring must be intelligent enough to identify the root cause of the model drift proactively and provide feedback. Dynamic ensemble selection (DES) determines which combination of the model’s combined result outperforms as compared to using a single model for prediction accuracy. Ensemble models can capture linear and non-linear relationships in the data. In a complex, unseen situation, depending on a single model is like touching and trying to identify an unknown (elephant) in a dark room.
Given that model decay is inevitable, monitoring models in isolation (silo manner) is time-consuming, resource-intensive and inefficient. Organizations must have centralized telemetry that monitors models, captures dependencies before model performance starts deteriorating, and reports in a timely manner. This contributes operational efficiency and scale, especially for organizations that have or expect to have hundreds of models. Data pipelines must support sustainable Continuous Integration (CI)/Continuous Deployment (CD) for speed, agility and repeatability to drive business value.
Tracking data and concept drift is extremely important to ensure that model monitoring is effective and accurately accounts for unprecedented events. Here are some best practices that companies can adopt for effective model monitoring:
- Centralize model monitoring with continuous tracking at a high-frequency basis (typically daily) in a systematic approach across the institution. This must be applied for all models in the inventory—recalibrating models based on annual model review or validation is too late.
- Establish ongoing monitoring through an automated process, such as a global monitoring dashboard with drill-down capability into any individual model, that can detect any unexpected behavior and communicate to the stakeholders. This can provide additional insights on correlations between model behavior.
- Continuously evaluate champion and challenger models and choose the model (or ensemble of models) dynamically that performs best based on the context.
- Identify early warnings in the model’s performance proactively, rather than reacting to issues that have already arisen and may be costly to remediate.
- Develop an aggregate metric that quantifies the overall model risk of the institution at any point in time and ensure that it’s within the model risk appetite set by the board of directors.
- Retrain models with the most recent data available to capture the trends; a first-in first-out (FIFO) data structure can capture the trend changes over time through adaptive learning. Data science pipelines have to be scalable to support CI/CD.
- Keep humans in the loop and employ expert judgment to ensure the results make sense.
For ongoing successful usage of the models, continuous monitoring of model performance and understanding of the context are critical. With these best practices, effectiveness and credibility will be enhanced at all times—even in uncertain times like these.
This post is a sequel to my previous article, “AI Modeling in the time of COVID-19.” Any comments and thoughts are welcome!
About the Author:
Raj Gangavarapu is Head of Data Science at Diwo, your intelligent advisor to turn AI into action. He has two decades of leadership experience helping companies to solve complex business problems by leveraging data and analytics. He is a speaker at various academic and industry conferences on data science, risk, Artificial Intelligence (AI) and Machine Learning (ML).